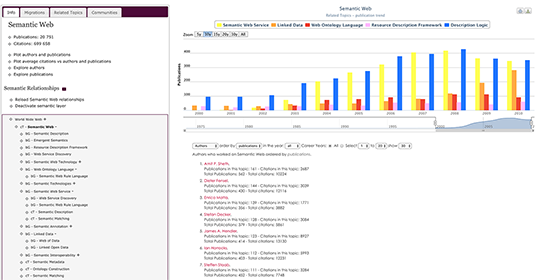
Klink-2 is an application which takes as input large amounts of scholarly metadata and automatically generates an OWL ontology containing all the research areas mined from the input data and their semantic relationships. It was developed to produced large scale ontology of research topics.
The traditional way to address the problem of identifying and structuring research topics has been to adopt human-crafted taxonomies, such as the ACM Computing Classification System. Unfortunately, these kinds of taxonomies suffer from several limitations. First, building a large taxonomy of research areas requires a large number of experts and is an expensive and lengthy process. Secondly, these taxonomies are very-coarse grained and usually represent wide categories of activity, rather than the fine-grained topics addressed by researchers. In the third instance, human-crafted taxonomies are subjective and depend on the understanding of the specific experts chosen to produce it. However, in this domain it is common for experts to disagree about the relationships between topics and be biased by their background.
Klink-2 addresses these limitations, since it automatically generates large-scale and very granular ontologies of research areas which can be easily kept up-to-date, by running Klink-2 on new data. The resulting knowledge base is completely data-driven, since it is produced directly from scientific literature and does not suffer from human biases.
Rexplore smart analytics and information extraction algorithms are powered by an ontology produced by Klink-2, which allows a very granular and comprehensive representation of research topics.
A Computer Science ontology produced by Klink-2 is used also by the Smart Topic Miner, the web application developed by the Rexplore team to support the Springer Nature Computer Science editorial team in classifying proceedings in the LNCS family.
You can try a public demo of STM at http://rexplore.kmi.open.ac.uk/STM_demo/
Relevant papers:
- Klink-2: Osborne, F. and Motta, E. (2015) Klink-2: Integrating Multiple Web Sources to Generate Semantic Topic Networks, International Semantic Web Conference 2015, Bethlehem, Pennsylvania, USA
- Klink (Previous version): Osborne, F. and Motta, E. (2012) Mining Semantic Relations between Research Areas, International Semantic Web Conference, Boston, MA